Building a Kubernetes Pod Doctor with Gradio and GPT-4
Hey devs,
As many of you, I’ve been exploring how AI can help me be more productive with my day to day tasks. So “Pod Doctor” was born. This is a little tool (just 180 lines!) which helps me with Kubernetes Pod troubleshooting faster than if I were to do it myself. You can find the full code on github and in this beginner tutorial we’ll go through the steps required to be able build similar tools for yourself.
We’ll learn how to create Gradio's chatbot UI, integrate the Kubernetes Python client, and leverage GPT-4's language for understanding and reasoning.

User Interface:
The user interface of the Pod Doctor application is built using Gradio, which is a Python library for creating customizable UI components and deploying machine learning models. The interface consists of the following elements:
- Title: A label displaying the name of the application, "Pod Doctor."
- Chatbot: A chatbot-style interface for displaying the conversation between the user and the language model.
- Namespace Dropdown: A dropdown menu that allows users to select the Kubernetes namespace they want to interact with.
- Pod Dropdown: A dropdown menu that populates with the list of pods available in the selected namespace.
- Include Events Checkbox: A checkbox that allows users to include pod events in the information provided to the language model.
- Include Logs Checkbox: A checkbox that allows users to include pod logs in the information provided to the language model.
- Message Input: A text input field where users can type their messages or queries.
with gr.Blocks(theme=gr.themes.Soft()) as demo:
with gr.Row():
title = gr.Label("Pod Doctor")
with gr.Row():
with gr.Column(scale=4):
chatbot = gr.Chatbot(height="600px")
with gr.Column(scale=1):
namespace = gr.Dropdown(
list_namespaces(),
label="Namespace",
info="Select the namespace you want to check the pod in.",
interactive=True,
)
pod = gr.Dropdown(
[],
label="Pod",
info="Select the pod you want to interact with.",
interactive=True,
)
include_events = gr.Checkbox(
label="Include Events",
info="Include the pod events.",
interactive=True,
)
include_logs = gr.Checkbox(
label="Include Logs",
info="Include the pod logs.",
interactive=True,
)
namespace.change(namespace_change, inputs=namespace, outputs=pod)
with gr.Row():
msg = gr.Textbox()
def respond(message, chat_history, namespace, pod, include_events, include_logs):
if not namespace or not pod or not message:
chat_history.append((message or "Error", "Please select a namespace and pod."))
return "", chat_history
bot_message = call_llm(message, namespace, pod, include_events, include_logs)
new_message = f"Namespace {namespace}, Pod: {pod}, Include Events: {include_events}, Include Logs: {include_logs} \n {message}"
chat_history.append((new_message, bot_message))
return "", chat_history
msg.submit(respond, [msg, chatbot, namespace, pod, include_events, include_logs], [msg, chatbot])
As we progress through the tutorial, you may encounter function calls that are not yet defined. However, these functions will be explained and implemented as we move forward.
Let's define a function that is responsible for updating the dropdown of pods once a namespace is selected:
def namespace_change(value):
"""
Returns a Dropdown widget populated with a list of pods based on the given value.
Args:
value (str): The namespace to filter the pods.
Returns:
gr.Dropdown: A Dropdown widget with choices populated from the list of pods.
"""
pods = list_pods(value)
return gr.Dropdown(choices=pods)
Kubernetes Integration
The Pod Doctor application integrates with a Kubernetes cluster using the kubernetes
Python library. The following functions handle the interaction with the Kubernetes API:
first we need to import the k8s library and load the config :
from kubernetes import client, config
config.load_kube_config()
list_namespaces()
: Retrieves a list of namespaces from the Kubernetes cluster.
def list_namespaces():
"""
Retrieves a list of namespaces from the Kubernetes cluster.
Returns:
A list of namespace names.
"""
v1 = client.CoreV1Api()
namespaces = v1.list_namespace()
return [namespace.metadata.name for namespace in namespaces.items]
list_pods(namespace)
: Lists all pods in the specified namespace.
def list_pods(namespace):
"""
List all pods in the specified namespace.
Args:
namespace (str): The namespace to list pods from.
Returns:
list: A list of pod names.
"""
v1 = client.CoreV1Api()
pods = v1.list_namespaced_pod(namespace)
return [pod.metadata.name for pod in pods.items]
get_pod_info(namespace, pod, include_events, include_logs)
: Retrieves detailed information about a specific pod, including events and logs if requested.
def get_pod_info(namespace, pod, include_events, include_logs):
"""
Retrieves information about a specific pod in a given namespace.
Args:
namespace (str): The namespace of the pod.
pod (str): The name of the pod.
include_events (bool): Flag indicating whether to include events associated with the pod.
include_logs (bool): Flag indicating whether to include logs of the pod.
Returns:
dict: A dictionary containing the pod information, events (if include_events is True), and logs (if include_logs is True).
"""
v1 = client.CoreV1Api()
pod_info = v1.read_namespaced_pod(pod, namespace)
pod_info_map = pod_info.to_dict()
pod_info_map["metadata"]["managed_fields"] = None
info = {
"PodInfo": pod_info_map,
}
if include_events:
events = v1.list_namespaced_event(namespace)
info["Events"] = [
{
"Name": event.metadata.name,
"Message": event.message,
"Reason": event.reason,
}
for event in events.items
if event.involved_object.name == pod
]
if include_logs:
logs = v1.read_namespaced_pod_log(pod, namespace)
info["Logs"] = logs
return info
LLM & GPT-4
The heart of the Pod Doctor application is the integration with the GPT-4 language model from OpenAI. The call_llm
function is responsible for generating a response based on the user's message, the selected namespace, pod, and the options for including events and logs.
Here's how the call_llm
function works:
- The
get_pod_info
function is called to retrieve the pod information, including events and logs if requested. - The
create_prompt
function generates a prompt message by combining the user's message with the pod information. - The OpenAI API is called using the
openAiClient.chat.completions.create
method, passing the prompt message and the GPT-4 model. - The response from the language model is returned and displayed in the chatbot interface.
from openai import OpenAI
import yaml
openAiClient = OpenAI()
def create_prompt(msg, info):
"""
Creates a prompt message with the given message and pod information.
Args:
msg (str): The main message for the prompt.
info (dict): A dictionary containing pod information.
Returns:
str: The generated prompt message.
"""
prompt = f"{msg}\n"
prompt += f"Pod Info: \n {yaml.dump(info['PodInfo'])} \n"
if info.get("Events"):
prompt += f"Here are the last few events for the pod: \n"
for event in info["Events"]:
prompt += f"Event: {event['Name']}, Reason: {event['Reason']}, Message: {event['Message']}\n"
if info.get("Logs"):
prompt += f"Here are the last few logs for the pod: \n"
prompt += info["Logs"]
return prompt
def call_llm(msg, namespace, pod, include_events, include_logs):
"""
Calls the Language Model to generate a response based on the given message, namespace, pod, and options.
Args:
msg (str): The user's message.
namespace (str): The namespace of the pod.
pod (str): The name of the pod.
include_events (bool): Whether to include events in the pod information.
include_logs (bool): Whether to include logs in the pod information.
Returns:
str: The generated response from the Language Model.
"""
info = get_pod_info(namespace, pod, include_events, include_logs)
prompt = create_prompt(msg, info)
completion = openAiClient.chat.completions.create(
model="gpt-3.5-turbo",
messages=[
{"role": "system", "content": "You are a kubernetes expert, and will help the user with request below based on the context and info provided"},
{"role": "user", "content": prompt}
]
)
return completion.choices[0].message.content
Usage
We need one piece of code to wrap everything together:
if __name__ == "__main__":
demo.launch()
export OPENAI_API_KEY='sk-xxxxxxxxxx'
python app.py
To use the Pod Doctor application, follow these steps:
- Make sure your local kubernetes config is setup try
kubectl cluster-info
- Select the desired Kubernetes namespace from the "Namespace" dropdown.
- Select the pod you want to interact with from the "Pod" dropdown.
- Optional: Check the "Include Events" and "Include Logs" checkboxes if you want to include pod events and logs in the information provided to the language model.
- Type your message or query in the text input field and press Enter.
- The application will display the response from the GPT-4 language model in the chatbot interface.
Let's try a deploying a pod with the wrong port in the probes configuration and ask Pod Doctor to troubleshoot it for us.
Below is the bad nginx pod we will apply:
apiVersion: v1
kind: Pod
metadata:
name: nginx
spec:
containers:
- name: nginx
image: nginx
ports:
- containerPort: 80
livenessProbe:
httpGet:
path: /
port: 81
readinessProbe:
httpGet:
path: /
port: 81
After we apply this yaml we go to our app, select the namespace where we aplied it, select include events and logs, and type `troubleshoot` in the textbox, hit enter and watch the magic:
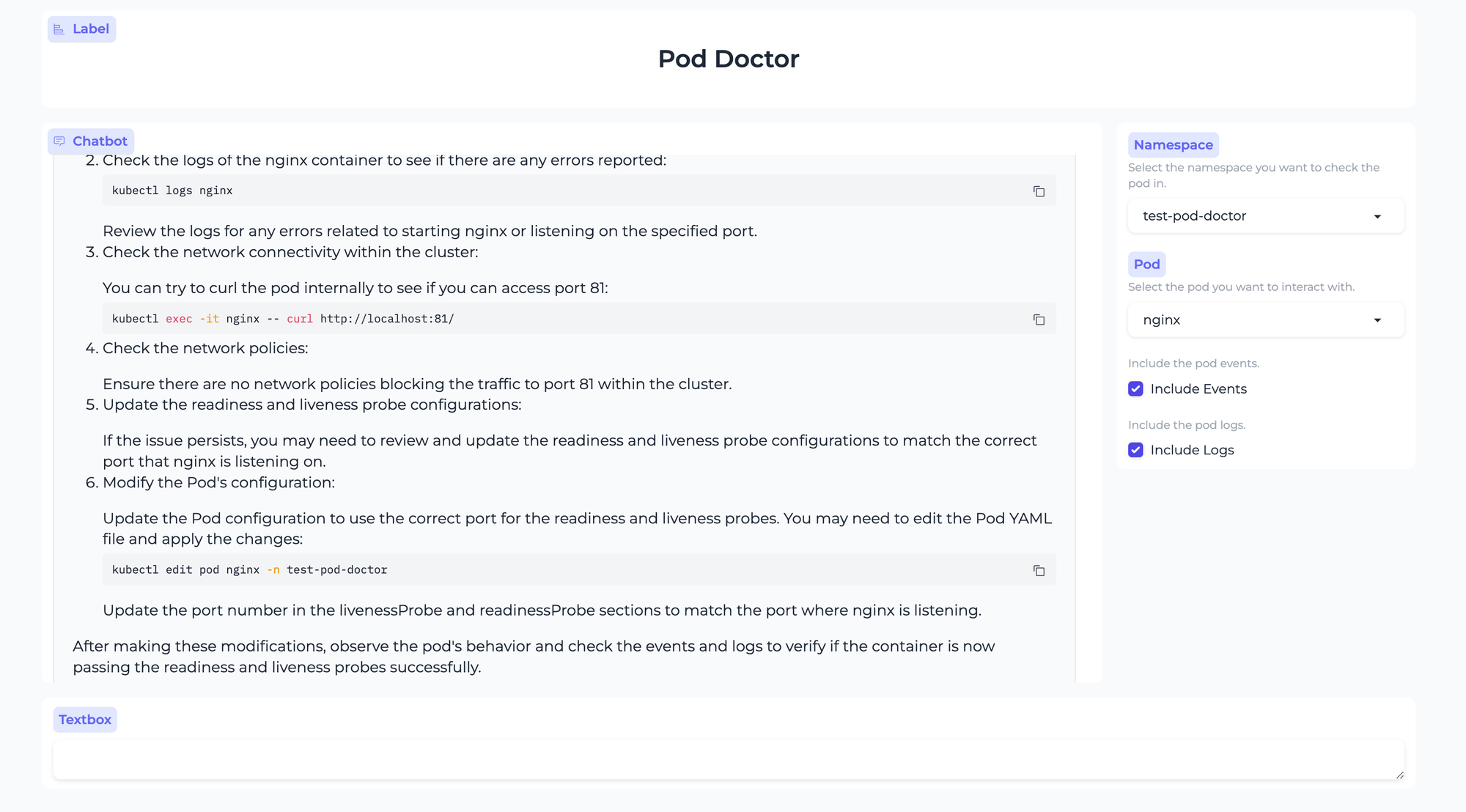
As we can see Pod Doctor was able to successfully troubleshoot the pod, and provide a root cause solution.
Conclusion
By combining the power of Gradio for building user interfaces, the Kubernetes Python library for interacting with Kubernetes pods, and the advanced language understanding capabilities of GPT-4, the Pod Doctor application provides a powerful and intuitive way to interact with and troubleshoot your Kubernetes pods.
You can find the full code in github.
Member discussion